Proving Payroll Compliance
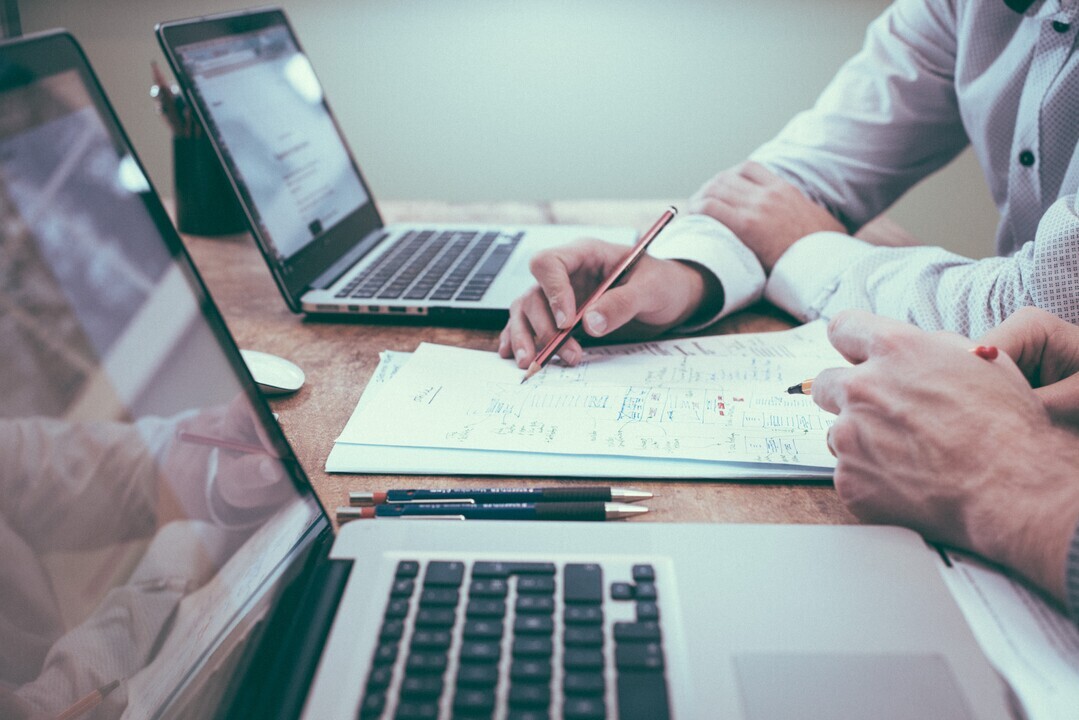
Proving payroll compliance and PaidRight’s data quality framework
The data we collect is the key in how we prove our compliance. If a problem does arise, we have to go back and look at what was planned to happen (rosters) and what actually happened (timesheets) and what was in place in order to make those calculations, and payments (pay records).
From a data quality perspective, the following sets a framework for collecting good quality payroll data to prove our compliance.
PaidRight’s business records data quality framework
Completeness — Are you collecting all the right data in accordance with the law?
There is guidance from legislation on the data that we are required to collect. The Fair Work Act requires businesses to keep records easily accessible for a Fair Work Inspector as well as the records of the last 7 years of operations.
The Fair Work Act requires us to keep several types of records including; general records, pay records, hours of work records, leave records and superannuation contribution records.
It also requires us to record; individual flexibility arrangement records, annualised wage arrangement records, guarantee of earnings records, termination records and transfer of business records.
There is a lot of data that we must collect in order to fulfil business record requirements as outlined in the Fair Work Act as well as specific modern awards and enterprise agreements. For more information on the data we are required to collect there is a great Fair Work Fact Sheet.
As most businesses are now using payroll systems to collect business records data, maintaining complete business records is not impossible but complete records do not necessarily mean you are compliant. There may still be invalid or illegal values in these data sets that could hinder our ability to prove compliance.
Accuracy — Is the data that we have collected accurate?
Accurate data reflects reality, however with the likelihood that numerous systems are being used as well as humans interacting with those systems data might not always be accurate. Some common examples we see of inaccurate records, that impact the accuracy of the data are;
- Duplicate shifts - meaning multiple shifts have the same employee and shift information or exact identical rows in your records.
- Duplicate data - meaning multiple records have the same values for all the following fields: Employee ID, Pay Period Start, Pay Sub Category, Pay Units, and Pay Amount
It’s important that the data collected is accurate because it can have an impact on the accuracy of your employees entitlements, but also makes it harder to understand if the duplicate records are real, or if the employee worked those hours. This will also have an impact on your ability to prove compliance.
Validity — Are the data and records valid? Are you collecting the right data points in the right places?
Validity is defined as the extent to which the data conforms to the expected format, type, and range. Some common examples we see of invalid records, that impact the accuracy of the data are;
- A particular shift is missing a start time
- A shift record is missing a finish time
- Shift records that show a start time after the end time
A valid shift should start before the end time, and they should have a valid start time and a valid end time. If these records are invalid, including them in your data set can impact your employee’s entitlement as well as make it difficult to understand and prove when that employee actually worked.
Consistency — Running multiple systems, means you have rosters, timesheets and pay records, are all these records consistent between all of those systems?
To effectively prove compliance, timesheet records must be consistent with pay records or you could potentially see;
- Missing records
- Timesheet hours worked that are inconsistent with hours worked on pay records
This is important because it may look like someone has worked and wasn’t paid for it or vice versa where an employee was paid but it looks like they haven't actually worked. This can be problematic when needing to prove compliance if a dispute arises.
Improving payroll data quality
From what we have seen, payroll data sets are very rarely perfect, which is partly the systems to blame and partly the people using those systems. Despite this, it’s become clear that the quality of data that we are collecting is one of the key contributors to any variance in pay; and your ability to prove that you are paying your employees correctly.
Getting payroll data right is one of the most important keys to unlocking insights when the need to prove compliance does arise.
