3 lessons we have learnt from working on the biggest remediations in the country
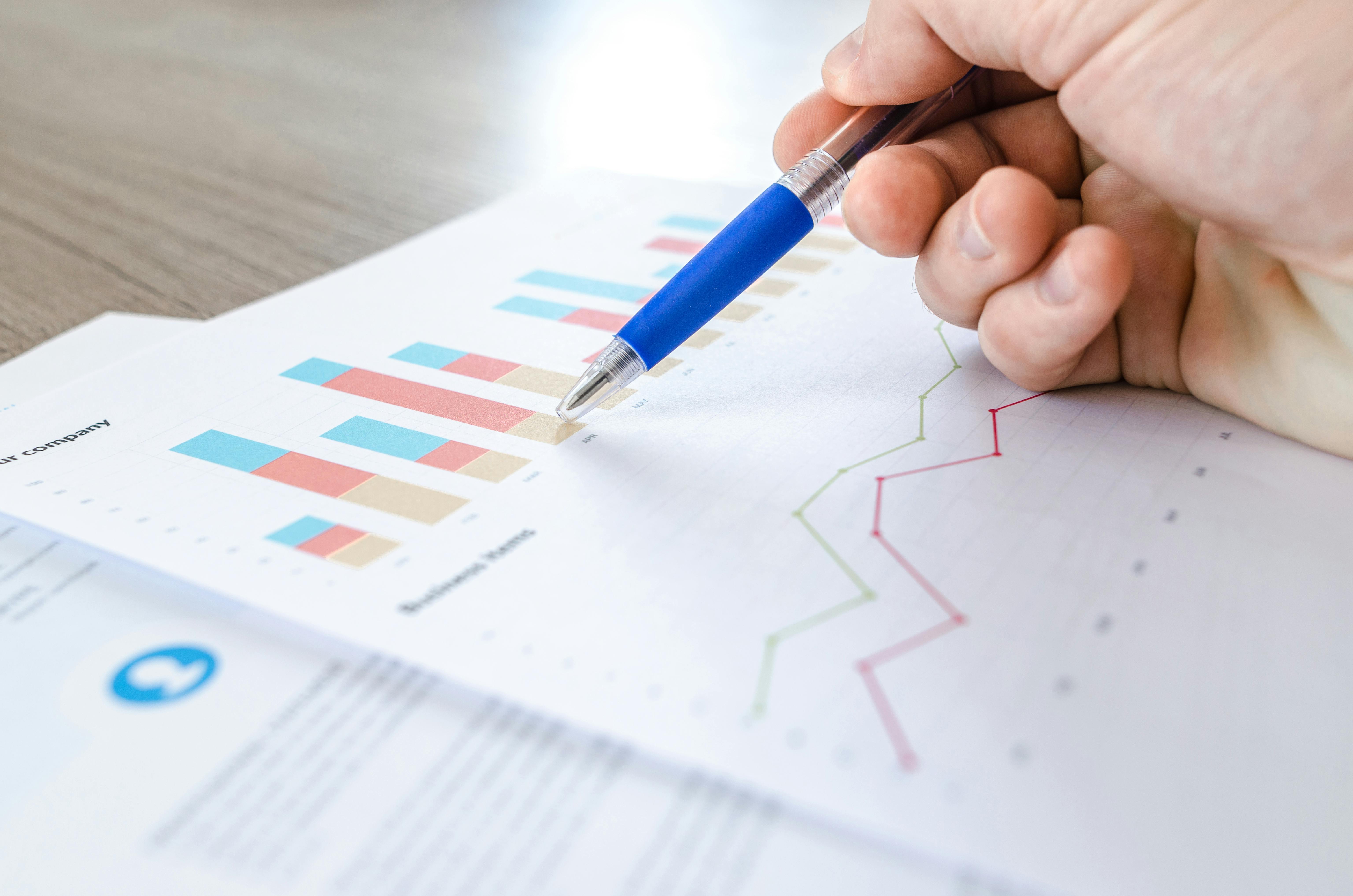
At PaidRight, we've tackled some of the largest and most complex wage remediation projects across various industries and business sizes. Our team has treated and analysed billions of rows of data.
Below are three key things we have learnt when it comes to dealing with complex data in wage remediation projects.
1. Wage remediation is a data issue, not an industry or legal one.
When it comes to wage remediation, the core challenge isn't tied to any specific industry. Instead, it revolves around data accuracy and representation of the actual work performed.
Typically, wage remediation is seen through a legal perspective, focusing on industry-specific awards, enterprise agreements, or regulations. A common sentiment we encounter is, "Our industry awards are far more complex than others."
However, this complexity is often overstated. In reality, about 40 common logic rules underpin most modern awards and enterprise agreements. While these rules have varying parameters and occasionally include unique clauses, the fundamental principles remain remarkably similar.
Another frequent claim is that an industry is unique. Yet, the data requirements for wage remediation are universal: we need information on the employee, their scheduled work hours, actual work hours, and their pay.
The data treatments are also consistent: Did the employee clock in and out? Did they take breaks? The specifics of the award or enterprise agreement don't alter these basic requirements. What matters is the data's ability to accurately represent the work performed.
Every industry has its nuances, and the logical framework within the legal instruments is vital for determining appropriate pay. However, the true challenge in remediation lies in managing and processing large, complex, and often incomplete datasets spanning several years.
2. Bad data doesn’t mean you can't do an effective remediation.
With so much emphasis on having precise data for accurate remediation, it's no surprise that data quality is a major concern for businesses when looking to do a remediation. However, there's good news: effective solutions exist to rectify even the most complex data sets, ensuring accurate wage remediation even when historical data is incomplete.
Data treatments for small gaps in data:
Historical data often fails to accurately reflect what actually happened in the business. Common data quality issues, such as missing values and overlapping entries, can falsely inflate or deflate the overall amount owed. These discrepancies must be meticulously addressed to ensure that any material adjustments are accurate.
The use of AI to process paper and scanned records:
We utilise AI technology to scan and digitise paper-based records, significantly enhancing the efficiency of remediation projects. In scenarios involving vast amounts of historical data, such as handwritten and typed timesheets spanning several years, the traditional manual process would demand extensive time and resources. However, by leveraging AI, we can dramatically accelerate this process.
Logic-based rules:
When data is missing from a certain time-period or data type, we apply logic-based rules to fill the gaps. For instance, if you are missing roster data for six months due to a system change, we can create model rosters based on existing rules, timesheets, or other data points. This method ensures continuity and accuracy in the absence of complete historical data.
3. The risks of Isolated focus
Another aspect that businesses encounter is the tendency to focus on one award or a section of the data without considering broader implications.
Focusing on a single award or section of payroll data might seem efficient, but it often leads to oversight and disparities, especially when changes are made to one group without considering the impact on others. For instance, making a treatment to your data based on one cohort of employees—such as clerks—may make sense for that specific group, but it could have unintended negative consequences when applied across other awards or cohorts.
When businesses isolate their focus to a specific award or subset of employees, they risk creating inconsistencies in payroll data. For example, an adjustment made to the data for clerks might optimise their pay calculations, ensuring compliance with their specific award. However, applying the same logic or treatment to another group of employees, such as warehouse workers or sales staff, who are governed by different awards, can lead to different issues that didn’t exist before the treatment was made.
